In 2020, the developing worry to see how AI functions and surveying the issue of inclination make the requirement for AI to be progressively logical and capable. The utilization of AutoML has welcomed a few advantages to information researchers on issues, for example, asset designing and calculation testing.
In 2021, it’s predicted an extraordinary expansion in the utilization of artificial intelligence and data warehouse in all subject matters, through the idea of hyper-automation, which includes a blend of numerous advances.
Data unification has been an impediment to efficient data processing for years. 80% of the time data scientists are reporting to find, unify and clean data from various sources before they can seriously start their analytical work. In this way, companies explore better ways of completing the process of data unification. Over the years, companies have made some progress in overcoming these issues, comprising the use of artificial intelligence (AI).
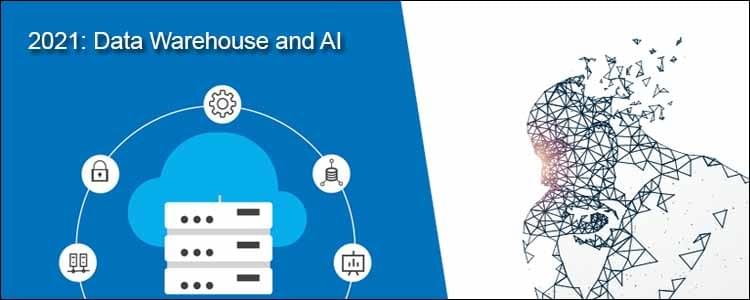
If there has been clear progress during the previous year, this was an improvement in the approval of cloud computing. Just pay attention to the actions taken with the exceptionally solid double-digit growth speed of one of the notable mists. For endeavors, it was tied in with adjusting to the virtual climate and compelled supply chains of an abruptly secured world.
A year ago (pre-covid), we looked at cloud adoption as a collection of logical steps, from DevTest to growth and re-platforming and conversion of core company back-end applications, and at the opportunistic adoption of new Saas infrastructure. However, with the retrospection, not suddenly, the headline of cloud acceptance over the past year was for companies to use cases to reflect on the new standard, where work and usage became more and more virtual and where traditional supply chains became pressured, the need to alter or create new services.
As firms move all the data infrastructure to a joined One motor scans various outlets, unbundled (computer storage separate from data lake) stacks, conventional data storage, and purely coupled database architecture assigned to legacy workflows. But one thing remains the same with this change – SQL is still the analytical lingua franca. For analytics, SQL can be used by data scientists, real-time data analytics consultants, product managers as well as their database administrators.
Artificial Intelligence – Demonstration of Machine
When corporations strive to reopen and produce enough income, they need to use intelligent technology to obtain critical knowledge in real-time to do so. Adopting artificial intelligence (AI) technologies can help businesses understand whether customer and employee strategies are working while stimulating growth. As businesses realize the unique potential of AI, we will see an increase in AI adoption rates across all industries to simplify business policy management and implementation, ensure safety, and improve the customer experience.
Going ahead, Responsible AI won't be another pattern in 2021. We do expect anyway that reestablished endeavors will be put resources into reasonableness attributable to the outer weight of guideline, mirroring the political atmosphere, particularly in North America and Western Europe, for making tech organizations more responsible. Besides, the goal lines for Responsive AI will continue to move with it, as AI grows more comprehensive, and with it, the interest for public examination continues to grow. The test is that compared to last year, we have not seen much improvement in the specified AI. We illustrated the difficulties of getting AI out of the Black Box with a view to 2020 a year ago and what we think about it in detail the barriers to AI have generally changed less than last year.
Chilling out at the Lakehouse
The data warehouse vs. data lake was the most discussed trend. In turn, the voice diminishes. Data warehouse developers cite the size of cloud-native frameworks and multimodal data backing to support the data lake variation. Opponents of data lakes resist this parameter, especially when you use data-intensive AI Models run, and open source solutions can create data lakes as efficiently as data stores.
The truth is that each data store and the lake has different strengths. Yeah, cloud data warehouses can go into the petabyte region now, but the obstacle is economical to most companies: data lakes are typically cheaper at that size. Then again, no matter how the inquiry engine is streamlined, data lakes depend on file scans and will never be as successful as providing tables for indexing, compressing, or filtering data.
Looking forward, the data warehouse, as a correlation data lake is not supposed to be redundant for the most part. Finally, the option will be guided by your developers. Database developers from Classic SQL would probably, want to use the relational database lake, while data scientists and developers using languages like Java and Python may favor data lakehouses if they are inherently skeptical. Many companies cannot determine between a data storage center, a data case and a data lake.